Machine Learning is one area of Artificial Intelligence that is growing in the healthcare industry. Healthcare organizations are leveraging Machine Learning for everything from discovering new treatments to predicting staffing needs. But what is Machine Learning? And what should nurses know about it? In this Nurse’s Guide to Machine Learning, we will summarize all of the key things nurses should know about this field.
We will not dive into the statistical analysis behind Machine Learning. But if you want to learn more about it Towards Data Science offers a great, comprehensive article on this topic.

What is Machine Learning?
Machine Learning is the ability for a computer to solve a problem for which it has not been specifically programmed. Machines learn in a similar way to people. Many of us learned key nursing skills by seeing an example, and then applying that example to others that are similar.
For instance, once we learn how to perform a dressing change for one stage 4 pressure ulcer, we are able to use a similar approach for dressing other stage 4 pressure ulcers. There will be some variation based on the patient and their needs. But the principles of assessing, cleaning, and dressing the wound are the same.
That is because we learn by recognizing patterns. Once we see an example of a type of wound, we can recognize others that are similar. With Machine Learning, machines recognize patters using examples too. This is different from programming a machine with specific rules. Instead of telling the machine what to know, the machines learn to find patterns in data.
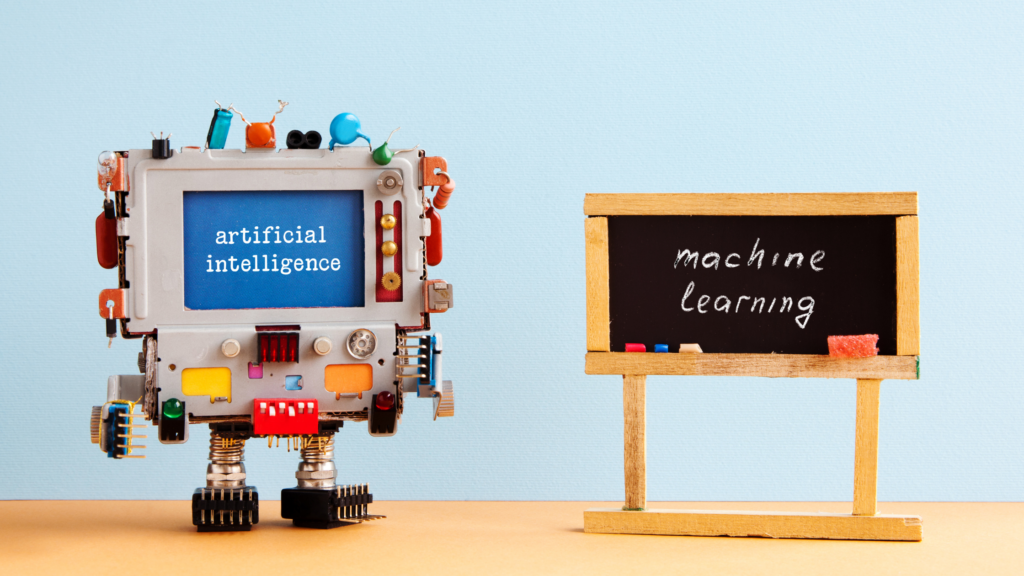
In machine learning, the machine is given a large data set to learn from. This data set could be a collection of labeled pictures, measurements from a group of samples, or characteristics of people with a specific condition. The machine then goes over the data repeatedly, learning what elements categorize items in the data set.
It is then given a fresh data set without the answers. For example, it may be given a set of unlabeled pictures and asked to identify what they contain, or given characteristics of a person and asked to identify their condition.
One goal of machine learning is to make future predictions. These are predictions such as where a virus will spread next, how many nurses will be needed on staff in December, or who should be screened for colon cancer early. In order to do that, the machine has to be able to learn without humans pre-programming it with the answer.

The Different Types of Machine Learning:
Machine Learning follows three basic steps:
- Taking in data
- Finding patterns in that data
- Predicting new patterns based on that data
For example, if a machine receives data about abnormal breast tissue, it can learn to identify cancerous cells. In fact, a recent study found that machine learning was able to detect breast cancer one to two years earlier than standard clinical methods.
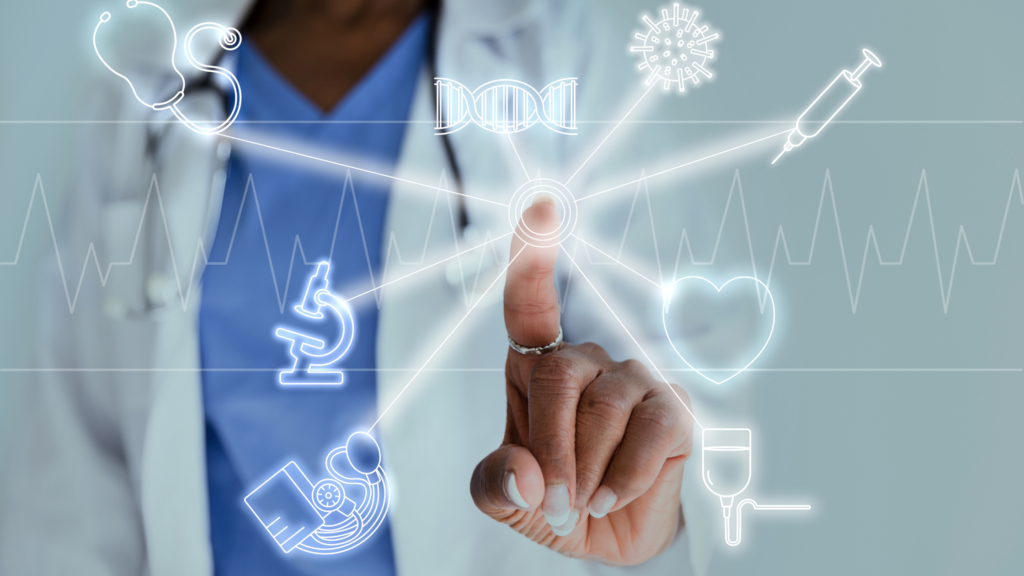
There are three types of Machine Learning you should know about:
Type #1: Supervised learning
This is where the machine receives labeled data For example, if you wanted a machine to classify patients into those who are pregnant versus those who are not, you could give it data with pregnant patients labeled. Then, if the machine receives a new set of data, it can easily recognize which patients are likely to be pregnant.
There is a story about how Target used its data to predict which customers were pregnant so they could start marketing diapers and baby clothes to them. However, they also accidentally alerted a teenager’s father that she was pregnant before she told him.
Type #2: Unsupervised learning
This is where the machine receives unlabeled data. The machine has to then find patterns on its own. The goal is for the machine to find patterns and group the data without help.
For example, if you wanted to better understand behavior of a cohort of type 2 diabetics, unsupervised learning might help. The machine can look for patterns and cluster data based on similarities it finds in certain groups. It might find that there are clusters of well-controlled type 2 diabetics who live within walking distance of a pharmacy.
Type #3: Reinforcement learning
This is where the machine receives a reward or penalty as it learns, which helps it to learn faster. You will find this type of learning in areas like playing complex games such as chess or Go, and is the type of learning behind self-driving vehicles.

How is Machine Learning Being Used in Healthcare?
There are many emerging ways healthcare organizations are applying Machine Learning. The list below is just a few of the numerous applications blooming in healthcare.
1: Population health
Because Machine Learning is good at identifying patterns, one natural fit in healthcare is with population health. In managing populations, healthcare companies are using Machine Learning for everything from reducing hospitalizations, to risk stratifying complex patients. For example, one hospital in New York used machine learning to predict patients most likely to develop sepsis. Another hospital in Pittsburgh uses it to predict which congestive heart failure patients are likely to end up in the emergency room.
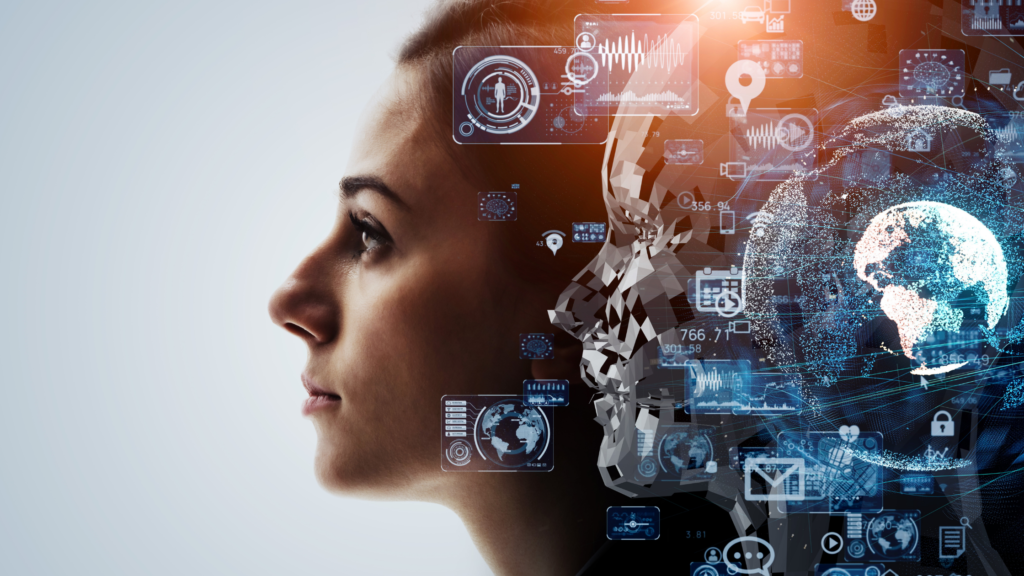
2: Medical imaging and diagnostics
Given the example of breast cancer detection given earlier, it should not be surprising that machine learning is becoming widely used in imaging and diagnostics. In addition to screening for cancers, some other areas of medicine where Machine Learning is helping with diagnosis:
- Identifying cardiovascular abnormalities – the goal is to automate detection of abnormalities and reduce errors, such as thickening of cardiac vessels or atrial enlargement.
- Detecting fractures – this can be especially helpful in identifying head and neck trauma in older adults after a fall.
- Earlier diagnosis of neurological diseases – this includes diagnosing neurological lesions and finding earlier biomarkers of disease like ALS.
3: Drug discovery
One application for Machine Learning is early-stage drug discovery, particularly with unsupervised learning where the answer is not already known. Machines can sift through massive amounts of data to find patterns that are hard or impossible for humans to detect on our own.
Pharmaceutical companies like Pfizer are using machine learning for research into different drugs. Pfizer partnered with IBM’s super computer, Watson, for cancer treatment research.
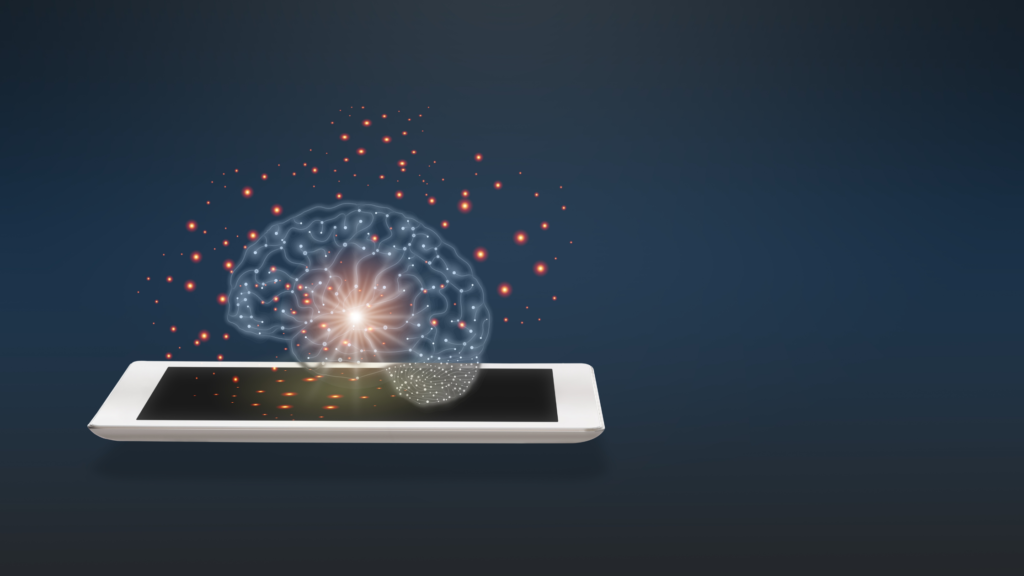
4: Staffing and scheduling
One of the uses that may impact nurses the most is in staffing and scheduling. This is one area where many healthcare organizations are investing. Because of staff shortages, the high cost of temps and travel nurses, and the stress on the system during the pandemic, this has become a hot topic.
Machine learning can help with staffing by creating more accurate forecasting, helping to automate scheduling, and adjusting schedules automatically instead of waiting for a human.

What Should Nurses Know About Machine Learning?
There are some key points nurses should know about Machine Learning as this technology spreads in healthcare.
1: Machine Learning looks backwards to look forwards.
“Machines can predict the future, as long as the future doesn’t look too different from the past.”
-Randy Lao, A Beginner’s Guide to Machine Learning
Machine Learning works by looking at past data, which is important to understand. As long as future data looks a lot like past data, Machine Learning can help to predict what will happen in the future. However, we have several recent examples of major events that rarely happen and are hard to predict. From the pandemic to the impact of climate change, black swan events can make it hard for machines to accurately predict what will happen next.
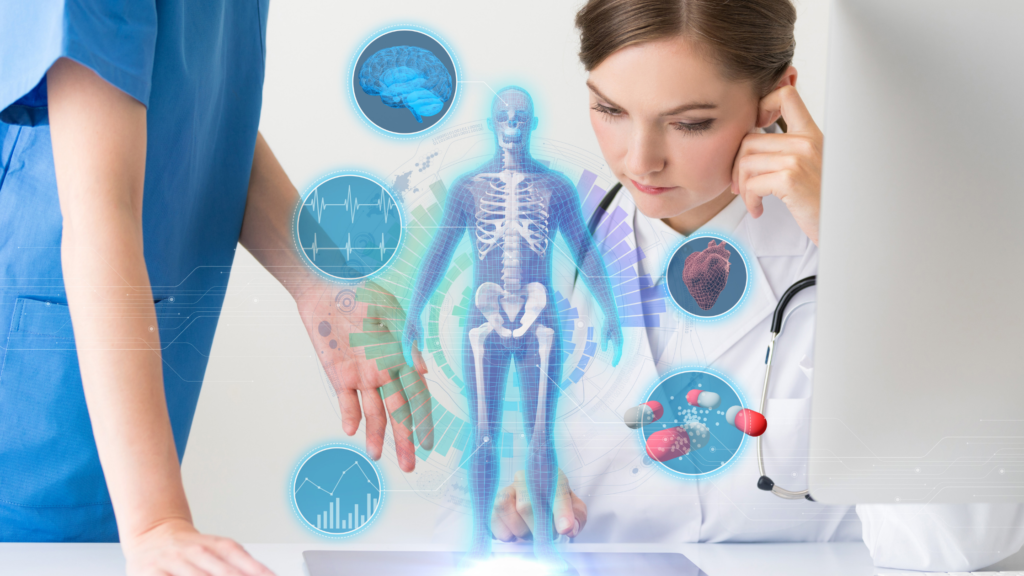
2: Machine learning is only as good as the data it receives.
That data is often created and collected by humans. This means the data can be subjective and even present bias. Bias does not have to be conscious or intentional for it to be present in data.
For example, if a hospital is located in a wealthier community and receives revenue from local taxes, it will likely have more resources for computer systems, equipment, and staff. Those additional resources might enable that hospital to collect more data on the health of the people in that community, which allows the hospital to be more specific in areas of focus.
On the other hand, a hospital in a poorer community may not have much tax revenue and access to the same level of resources. That impacts the hospital’s ability to collect data, and address health concerns in the area.
3: There is still a lot of untapped potential for Machine Learning.
In many ways, we are still in the early days of Machine Learning. However, investment into healthcare technology exploded in the last several years. It is very likely we will see Machine Learning leveraged for many more applications in the future.

Key Takeaways:
In this Nurse’s Guide to Machine Learning, we covered the basics of what Machine Learning is, uses in healthcare, and what nurses should know about it. The idea that machines can learn without programming is a powerful one. As healthcare finds new ways to apply this technology, it is important for nurses and other healthcare practitioners to understand it. There is still much untapped potential.

Like this topic? You may have what it takes to be a great Informatics Nurse! Here are two ways to get started:
Option 1: Sign up for Career Coaching! If you would like hands-on help planning your Nursing Informatics career, apply for one of my career coaching packages or book a Coaching Call. Check out my Services page for more information.
Option 2: Check out From Bedside Nurse to Informatics Nurse: A How-To Guide available in our online store.
0 Comments